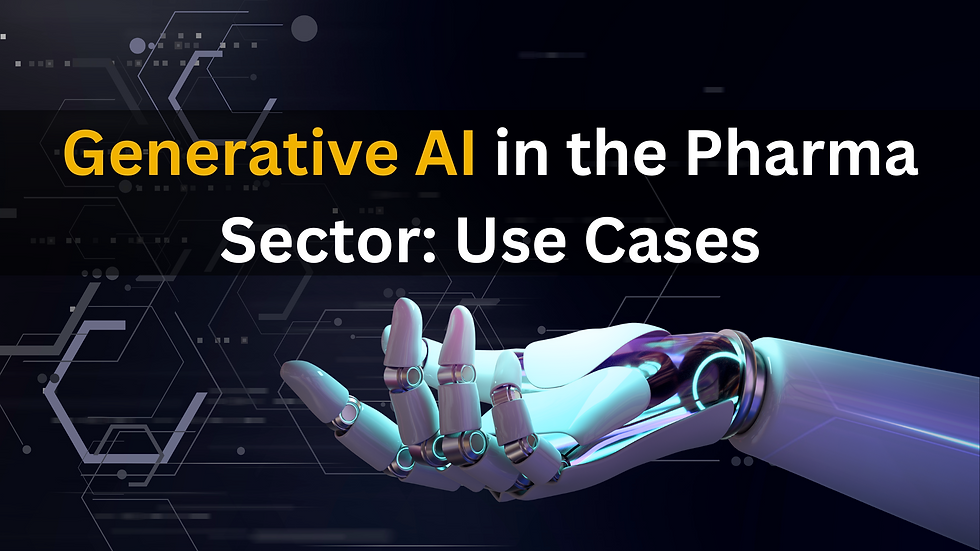
Generative AI has made a significant impact on the medical-product and pharma industries - from 3 to 5 percent of total annual revenue. Many pharmaceutical companies have long been using artificial intelligence. Researchers were applying AI models to understand the mechanism of diseases. For example, all use deep learning to understand the protein's structure, transforming their understanding.
Gen-AI could create value across the business value chain because of its ability to grope various data sources - structured or unstructured. Foundational models are based not only on languages but also on images, visuals, patient information, and other data.
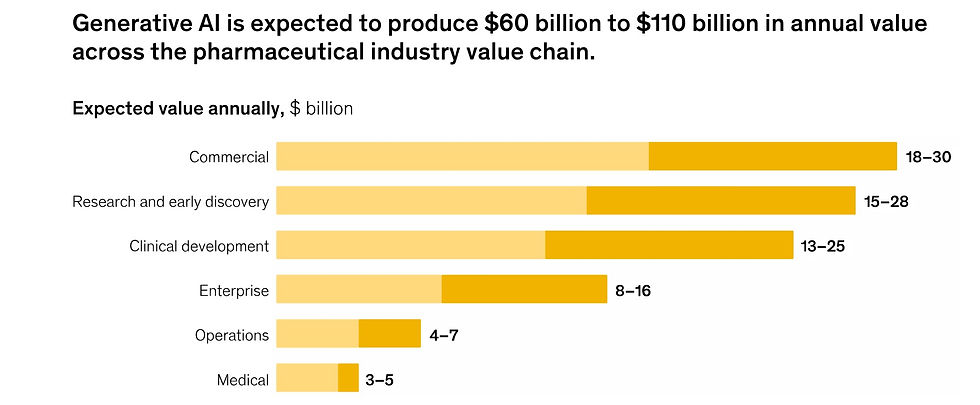
Generative AI in Medical and Pharma Products
Medical discovery includes streamlining the possible compounds to those that could treat the specific conditions. The ability of Generative AI to process immense amounts of data can facilitate output across multiple use cases:
Most specific use cases in under four categories: knowledge extraction, customer engagement (services for patients and healthcare providers), content generation (such as generative chemistry), and software generation. Companies should move quickly to grab the competitive edge. Also, one must understand that Gen-AI cannot be an outlying initiative, it should be a priority.
Now let's dive into specific use cases of generative AI in pharma and medical products.
Extracting scientific knowledge
Researchers spend much time extracting information from various documents like scientific publications, trial data, and patents to understand disease and drug targets. This is not only onerous but often gives inaccurate or incomplete information. GPT-empowered knowledge extraction tools can analyze unstructured data, including text, images, and other types of information can reduce this burden. Unlike traditional solutions based on natural language processing (NLP), new AI tools give a much broader understanding of the medical context. Researchers can create open-ended FAQs, and integrate additional evidence with prompt engineering. Little training would be required to tailor information for specific use cases.
Potential impact: More than 30% increase in manual assessments of drug targets
Trial performance co-pilot
Generative AI can rapidly analyze vast amounts of structured and unstructured data. Therefore, it is a powerful study-team companion, which shares insights and proposes interventions to refine the clinical trials outcomes. Some pharmacies have already created analytics platforms that support decision-making during clinical development by giving insights to speed up clinical trials.
Such AI co-pilots, facilitate the trials by empowering the study team in 3 important ways: conversational AI, actionable insights in an appealing format, early interventions; and automated communication drafts. These tools also promise to pace enrollment by automating analyses and addressing enrollment changes.
Potential impact: Estimated 20% cost efficiency improvement; 10 to 20% faster enrollment
A Gen AI assistant for manufacturing
Gen AI-powered virtual assistance can optimize medicine manufacturing by instantly suggesting relevant operating procedures and guiding for repeatable right-first-time operations. It also, helps supervisors manage and monitor performance in real time. Virtual assistants allow predictive maintenance - preventing shutdowns by flagging potential line failures, automating intervention generation, troubleshooting plans and maintenance tickets, and improving repair and replacement schedules.
Potential impact: Approximately 12 to 15% improvement in OEE (overall equipment effectiveness) by lowering key losses. More than a 30 percent increment in productivity for line leaders. About 15 to 35% work burden reduction for technicians, and a 5% reduction in quality cost
Customer-enablement co-pilot
Sales reps, marketers, and the customer-facing team have an unequaled amount of information at their disposal. However, they often lack in synthesizing the right information at the right time. Thus, to increase the productivity of reps, gen AI tools are being developed.
Chatbots and virtual assistants provide on-demand summarization, retrieval, and synthesis of structured and unstructured data. This makes campaigns more efficient and enables tailored and targeted conversations between sales reps and care providers.
Potential impact: About 10 to 15% improvement in the effectiveness and productivity of a customer-facing team, leading to 1-2% topline growth
Optimizing the customer experience
Most patients do not take their prescribed medications or never fill the prescriptions in the first place. For example, about 50% of the patients stop taking new medications during the first year. This increases often among patients who benefit from therapies, also because of the complexity of getting reimbursed for the prescriptions of new medicines. Gen AI can fix this problem by providing physicians and patients with on-demand insight into reimbursement and care options.
Gen AI can also help elevate critical issues to experts while delegating patient and physician's offices with a wide range of self-service tools. All of this led to improved patient conformance and outcomes since the technology can meet unaddressed needs by upskilling patient service teams.
Potential impact: About 5-10% reduce the number of patient drop-offs by providing a better customer experience
Conditions selection for asset strategy
Knowledge extraction capabilities of Gen-AI can help researchers identify which condition to target with a specific drug - the most important decision facing. To make this decision, researchers must gather information from various sources like literature reviews, opinion leaders, trial data, and competitor activities. Condition selection often only covers part of the available evidence, so a conclusion may not be ideal. Generative AI can help address this issue by determining a wide range of unstructured data sets.
Potential impact: Successfully prioritizing indications that have gone for approval in clinics; abolishing those that would probably fail
Smart data management
Today, data management is an intensive process, requiring manual trial configuration of an electronic data capture system and a thorough review and placating of new patient data. By collaborating with Gen AI and traditional capabilities, it is easy to automate data management across multiple steps.
Database creation is possible with one click based on the protocol, patient profile, and visit type. Data then can be reviewed clean automatically in real-time and queries efficiently and intelligently auto-generated based on patient status, trial context, and site actions - focusing on critical data gaps to be considered before database lock.
Potential impact: More than 30% cost saving on data management; 50% time reduction in database lock; 70% fewer manual queries
Augmented sourcing
The procurement process is time-consuming and inefficient. Category managers should analyze and research market trends, pricing data, and supplier information before evaluating RFPs. A gen AI bot can enhance the process by creating the first RFP draft, invoices, and purchase orders.
The AI solution can also assist negotiators in finding relevant past negotiation patterns and outcomes. Once the supplier is on board, Gen AI facilitates smart contract management and monitors supplier performance in real-time.
Potential impact: Procurement management expenses reduce by up to 5 to 10%; productivity gains (depending on categories and roles) by 50 to 80%
Summaries of the scientific and medical literature
One more challenge for medical affairs teams is appealing to external stakeholders with scientific content customized specifically for their own needs. This content's technical nurture and demand to comply with regulatory standards means that MSLs often provide stakeholders with long scientific documents like presentation decks or research papers. Although they prefer more focused responses, like the answer to a specific query.
Using Gen-AI-empowered tools trained on multiple documents, the medical-affair team can pull together tailored material, including text, videos, data tables, infographics, and audio.
Potential impact: A 2-3 times rise in engagement, associated with personalized content in other industries. Also among initial adopters in medical affairs
Sharper, more efficient medical communication
Generative AI can significantly reduce the time required to design scientific and technical documents, by facilitating the composition of medical information request responses. In addition to content generation, gen AI tools can help review and modify it, improving productivity and enhancing workflows. For example, medical professionals are often required to respond to emails from clinicians asking for details on clinical trials.
At present, this time-consuming task often requires various people to understand the question, identify the literature, and synthesize responses. Generative AI helps screen upcoming requests, abstract relevant content, and even create a tailored first draft of an answer. So, medical teams can respond immediately, using a wider base of scientific evidence.
Potential impact: Up to 20-30% savings in medical writing costs and 50-70 percent time reduction in delivering medical legal advice
Medical and legal review automation
The medical and regulatory process is undertaken to ensure all the promotional and advertising content is accurate and adheres to regulatory requirements. Today, MLR reviewers manually review content, address new claims, identify paradigms, and track similarities across different types of data sources. Gen AI tools can streamline the entire process in many ways: tracking the reuse of previously approved references and materials, reviewing new materials for potentially problematic language, accelerating compliance checks, and pacing up the approval process by ensuring that reviewers stay in the arc.
Potential impact: Acceleration of the content approval process by 2-3%, an increase in the review pace
Factors for medical and pharma products organizations to consider
Organizations before deploying generative AI into operations, medical executive teams must be aware of factors that can limit their to capture its benefits:
Explainability - The absence of transparency to the origins of generated content and traceability of data sources could make it difficult to update the model. For example, generative AI design for synthesizing scientific literature is not able to point to the specific article that led it to deduce a new treatment popularity among physicians. The technology can create inaccurate or incomplete responses for the given context. Thus, the model needs to be trained to point to specific articles or data sources, and then involve humans for checking.
The human need in the loop - Organizations are required to implement a quality check on operations that shifts from human agents to conversation AI. For example, reps-generated emails, and detailed quality checks on AI-assisted operations. The increasing need to verify whether generated content is fact and interference-based raises the need for a new control quality level.
Privacy considerations - The use of medical records and clinical images could increase the concern that information will leak. Thus pharma companies are required to protect patient privacy and regulations.
Commentaires